How AI is Enhancing Data Categorization in E-Discovery
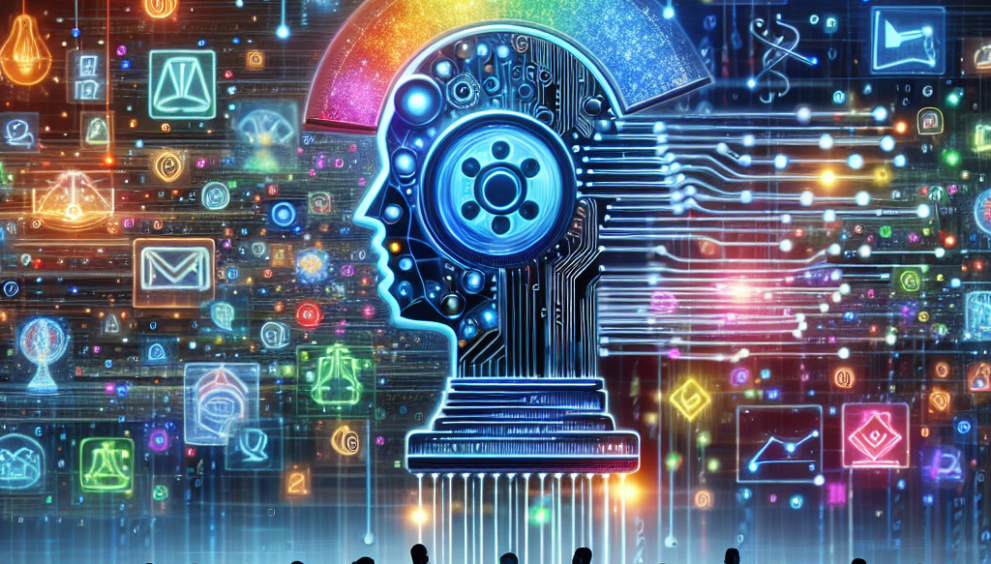
Table of Contents
- Introduction
- Improved Accuracy in Document Classification
- Automated Data Tagging and Metadata Extraction
- Predictive Coding and Machine Learning Algorithms
- Enhanced Search Capabilities with Natural Language Processing
- Streamlining Review Processes with AI Tools
- Reducing Costs and Time in E-Discovery Workflows
- Conclusion
Introduction
Artificial Intelligence (AI) is revolutionizing the field of e-discovery by significantly enhancing data categorization processes. As organizations grapple with the exponential growth of digital information, traditional methods of data sorting and analysis have become increasingly inefficient and time-consuming. AI technologies, particularly machine learning and natural language processing, enable legal teams to automate the categorization of vast datasets, improving accuracy and speed. By intelligently identifying relevant documents, flagging privileged information, and streamlining the review process, AI not only reduces costs but also enhances the overall effectiveness of e-discovery efforts. This transformation is crucial in today’s data-driven legal landscape, where timely and precise information retrieval can make a substantial difference in case outcomes.
Improved Accuracy in Document Classification
In e-discovery, legal teams face the challenge of sifting through vast amounts of data. The integration of artificial intelligence (AI) has become a game changer, especially in document classification. By using advanced algorithms and machine learning, AI enhances the accuracy of categorizing documents, making the e-discovery process more efficient and reducing the workload for legal professionals.
Key Points:
- High Volume of Data:
- Legal contexts generate enormous amounts of data, making manual review overwhelming and time-consuming.
- Traditional classification methods are often slow and prone to human error.
- Speed and Scale:
- AI can process thousands of documents quickly, identifying patterns and relationships that might be missed by human reviewers.
- This allows for a more thorough examination of data, ensuring relevant documents are not overlooked.
- Natural Language Processing (NLP):
- AI uses NLP to understand the context and meaning of documents.
- It can distinguish between similar terms and phrases, enhancing classification accuracy and identifying key evidence important to cases.
- Continuous Learning:
- Machine learning algorithms improve over time by training on historical data.
- As AI encounters new documents, it learns and refines its classification techniques, becoming better at identifying relevant information.
- Consistency:
- Human reviewers may interpret documents differently, leading to inconsistencies in classification.
- AI applies uniform criteria to all documents, ensuring consistent categorization, which is crucial in high-stakes legal situations.
- Cost and Efficiency:
- Improved accuracy reduces the time spent on manual document classification, lowering costs associated with prolonged review processes.
- Legal professionals can focus on strategic tasks, like developing case strategies and engaging clients.
Automated Data Tagging and Metadata Extraction
In e-discovery, the massive amount of data generated in legal contexts can be overwhelming. To help manage this data, artificial intelligence (AI) has become a transformative tool, particularly in automated data tagging and metadata extraction. By using advanced algorithms and machine learning, AI enhances the efficiency and accuracy of data categorization, making the e-discovery process smoother.
Key Points:
- Challenge of Data Volume:
- Legal teams face the daunting task of sorting through vast amounts of documents and emails.
- Traditional manual processes are time-consuming and prone to human error.
- Automated Data Tagging:
- AI-driven automated tagging significantly reduces the need for manual categorization.
- AI systems analyze documents quickly, identifying key terms and contextual clues that indicate relevance.
- This allows legal professionals to focus on strategic tasks, like case development and trial preparation.
- Improving Accuracy Over Time:
- AI systems learn from previous tagging decisions, adapting to the specific needs of each case.
- This continuous learning enhances tagging precision and aligns with the legal team’s expectations.
- The risk of missing critical information is minimized, leading to more effective e-discovery.
- Role of Metadata Extraction:
- Metadata provides essential information about documents, such as creation date, author, and modification history.
- Manually extracting metadata from large datasets is challenging, but AI automates this process.
- This allows legal teams to gain insights into the context and origin of the data.
- Uncovering Patterns:
- AI-driven metadata extraction can reveal connections between different pieces of evidence.
- By analyzing metadata, AI can identify potential leads or inconsistencies that are important in legal cases.
- This depth of analysis enables legal teams to make better-informed decisions.
- Overall Benefits:
- AI streamlines the categorization and analysis processes, saving time and resources.
- The integration of these technologies elevates the quality of legal work.
- Embracing AI solutions is becoming essential for success in the legal field.
Predictive Coding and Machine Learning Algorithms
In e-discovery, the massive amount of data generated during legal cases can be overwhelming. To manage this complexity, organizations are increasingly turning to artificial intelligence (AI), particularly predictive coding and machine learning algorithms. These technologies streamline data categorization and improve the accuracy and efficiency of legal document reviews.
Key Points:
- What is Predictive Coding?
- Predictive coding is a type of machine learning that helps identify relevant documents by learning from previously reviewed data.
- It starts with a small set of documents that legal professionals manually categorize, creating a training set for the algorithm.
- How It Works:
- The algorithm analyzes the training set to recognize patterns that distinguish relevant documents from irrelevant ones.
- Once trained, it can apply this knowledge to a larger dataset, reducing the time and effort needed for human reviewers.
- Efficiency Gains:
- By automating initial document review stages, predictive coding allows legal teams to focus on the most important materials.
- This optimization of resource allocation leads to more efficient workflows.
- Continuous Learning:
- Predictive coding improves over time through feedback from reviewers.
- As reviewers assess more documents, their feedback helps refine the algorithm’s understanding of relevance, adapting to the case’s nuances.
- Role of Machine Learning Algorithms:
- Machine learning algorithms analyze large datasets to uncover patterns and trends that may not be obvious to human reviewers.
- Techniques like natural language processing (NLP) allow machines to understand context, sentiment, and intent behind documents.
- Improved Document Categorization:
- These capabilities enable more nuanced categorization, helping to distinguish between similar documents and enhancing the overall quality of the review.
- Reducing Human Error:
- Traditional manual reviews can lead to inconsistencies and oversights, especially with large data volumes.
- Machine learning algorithms provide a consistent evaluation framework, enhancing the reliability of the review process.
- Empowering Legal Teams:
- By combining predictive coding and machine learning, legal teams can make informed decisions based on comprehensive data analysis.
- This empowers them to navigate the complexities of e-discovery with greater agility and precision.
You May Also Like: Cloud Computing’s Impact on E-Discovery and Data Storage
Enhanced Search Capabilities with Natural Language Processing
In e-discovery, the vast amount of data generated in legal contexts can be daunting. Traditional search methods often lead to inefficiencies and higher costs. However, artificial intelligence (AI), especially through natural language processing (NLP), is revolutionizing how legal professionals search through information. NLP enables more intuitive and context-aware searches, making the e-discovery process faster and more accurate.
Key Points:
- What is Natural Language Processing (NLP)?
- NLP is a technology that allows machines to understand and interpret human language meaningfully and contextually.
- It is particularly useful in e-discovery where language nuances can greatly affect document interpretation.
- Limitations of Traditional Keyword Searches:
- Traditional searches often rely on exact matches, leading to many irrelevant results.
- This method may overlook important documents that don’t contain the exact search terms.
- Enhanced Search Capabilities with NLP:
- NLP algorithms can understand synonyms, variations in phrasing, and even the intent behind a search query.
- This means that when a legal professional searches, the AI can return results that are relevant and contextually aligned with the legal issues.
- Improved Data Categorization:
- NLP enables categorization based on document content rather than just metadata (titles or tags).
- By analyzing the text, AI can identify themes, sentiments, and relationships, leading to more effective categorization.
- Adaptive Learning Over Time:
- Machine learning algorithms can learn from user interactions, refining search results with continued use.
- This means that the more a legal team uses the system, the more tailored and precise the search outcomes become.
- Time and Quality Benefits:
- Improved search capabilities save time, allowing legal professionals to focus on strategic decision-making rather than data retrieval.
- This enhances the overall quality of the review process.
- Fostering Collaboration Among Teams:
- With AI managing data categorization, legal teams can engage in deeper discussions about the findings.
- This collaborative environment helps in developing more informed strategies and decisions.
- Future Implications:
- The integration of NLP into e-discovery tools is a significant advancement in legal data management.
- As legal professionals adopt these technologies, they can expect improved efficiency, accuracy, and collaboration, leading to better legal outcomes.
Streamlining Review Processes with AI Tools
In e-discovery, the large amount of data generated in legal cases can be overwhelming. Traditional methods for sorting and reviewing this data often lead to inefficiencies and higher costs. However, artificial intelligence (AI) is changing this landscape by streamlining review processes and enhancing the effectiveness of e-discovery. By using advanced algorithms and machine learning, AI tools help legal professionals manage data categorization faster and more accurately.
Key Points:
- Automation of Document Categorization:
- AI tools can automate the categorization of documents, significantly speeding up the review process.
- This automation reduces the need for time-consuming manual reviews and minimizes the risk of human error.
- Advanced Language Understanding:
- AI utilizes natural language processing (NLP) to understand the context and nuances of language in documents.
- Unlike traditional keyword searches, which may miss synonyms or related terms, AI can grasp the underlying meaning, ensuring relevant information is not overlooked.
- Improved Accuracy:
- By analyzing documents contextually, AI tools enhance the accuracy of reviews.
- This leads to a lower risk of missing essential evidence in legal cases.
- Document Prioritization:
- AI can prioritize documents based on their relevance using predictive coding.
- The system learns from the decisions made by legal professionals, enabling it to better predict which new documents are important. This helps teams focus on the most pertinent materials first.
- Fostering Team Collaboration:
- AI tools provide real-time insights and analytics, which enhance collaboration among legal teams.
- Team members can share findings and strategies more effectively, leading to informed decision-making and a comprehensive approach to data categorization.
- Adapting to Evolving Legal Landscapes:
- As legal processes evolve, AI’s role in e-discovery becomes increasingly crucial.
- Legal professionals who use AI tools improve their efficiency and position themselves to better handle modern litigation challenges.
Reducing Costs and Time in E-Discovery Workflows
E-discovery, the process of identifying and reviewing electronic data for legal cases, can be time-consuming and costly. Traditionally, this process involved extensive manual review, leading to inefficiencies and the potential for human error. However, the integration of artificial intelligence (AI) is transforming e-discovery, enabling significant reductions in both costs and time.
Key Points:
- Speed and Accuracy of AI:
- AI algorithms can analyze and categorize data much faster and more accurately than humans.
- Machine learning allows AI to learn from previous cases, adapting to the specific needs of current projects.
- Streamlined Review Process:
- AI identifies patterns and relationships within data that may not be immediately apparent.
- Legal teams can concentrate on the most relevant information, enhancing overall efficiency.
- Reduced Time for Data Categorization:
- Traditional document reviews could take weeks or months.
- AI quickly processes large volumes of data, flagging relevant documents and discarding irrelevant ones, significantly shortening timelines.
- Cost Savings:
- Traditional e-discovery requires hiring large teams for document reviews, leading to high legal fees.
- AI reduces the need for extensive manpower, lowering overall costs.
- Increased accuracy from AI minimizes the risk of costly mistakes due to human oversight.
- Strategic Legal Practice:
- AI enables quicker identification of key documents and insights, allowing for informed decision-making.
- Legal teams can assess their cases earlier, improving preparation and negotiation strategies.
- Indispensable Role of AI:
- As the legal landscape evolves, AI’s role in e-discovery is becoming essential.
- Embracing AI enhances operational efficiency, reduces expenses, and improves client service.
Conclusion
AI is significantly enhancing data categorization in e-discovery by automating the identification, classification, and organization of vast amounts of electronic data. Through advanced algorithms and machine learning techniques, AI can quickly analyze documents, emails, and other digital content, improving accuracy and efficiency in the review process. This not only reduces the time and cost associated with e-discovery but also minimizes human error and enhances the ability to uncover relevant information. As AI continues to evolve, its role in e-discovery will likely expand, leading to more sophisticated categorization methods and better overall outcomes in legal investigations.